AugmentedEF
Using Augmented Reality to Enhance Attention in STEM Learning for Students with Executive Function Disabilities
Lead Staff:
Zac AlstadProject Staff:
Ibrahim Dahlstrom-HakkiJodi Asbell-Clarke
Kim Descoteaux
Kelly Paulson
Project Summary
The primary goal of this project is to support the foundational capabilities for STEM for post-secondary learners with attention deficits and executive function (EF) needs. AugmentedEF uses emerging technologies to explore novel and accessible tools for teaching and learning.
EF represents a broad skill set that is central to STEM learning and academic success, including skills related to attention, persistence, emotion regulation, and inhibition control (Barkley, 2012). The marginalization of learners with attention and EF differences occurs throughout schooling, and is a further cause of rampant inequities in our education system. There is an increased prevalence of attention to EF issues such as ADHD in many marginalized communities, including communities of color (Miller, et al., 2009), low SES communities (Russell et al., 2016) and communities of English language learners (Liontou, 2019).
Show More
Show Less
Given the demands placed on students in online learning contexts from the response to the COVID-19 pandemic, inequities due to EF issues have been brought to the forefront. Students with EF difficulties tend to underperform in independent work environments, like those required in many remote learning scenarios (Dahlstrom-Hakki et al., 2020), thus demands in digital environments disproportionately and negatively impact students with EF issues (Carter et al., 2020). While there are efforts to develop assistive technology to supplement a student’s organizational capabilities, many tools are expensive and exclusionary, and most rely on students’ ability to organize or initiate their own task monitoring (e.g., the use of a planner).
AugmentedEF presents a novel approach to this complex problem by developing an open-source learning tool that uses an augmented reality (AR) interface with a backend machine-learning architecture able to initiate and adapt task monitoring prompts based upon students’ perceived behaviors. AugmentedEF will be designed and tested with a broad audience of neurodiverse undergraduate students to examine and improve its efficacy as a STEM learning tool.
Research Activity
This tool represents a novel contribution to both the computer sciences and the learning sciences. AugmentedEF will develop an open-source tool based on machine learning algorithms that provides appropriate feedback to students related to their own level of focus and distractibility. The use of machine learning is an important feature because ultimately, it will not require a human to code off-task behavior.
There have been some initial attempts to look at user attention from the perspective of machine learning (Szafir & Mutlu, 2012) and others have attempted to explore prototypical ADHD behavior through the lens of machine learning tools (Duda et al., 2016). AugmentedEF will explore new algorithms and prompting tools that are useful to the field of EF support research.
Impact
The creation and validation of AugmentedEF is intended to have wide-reaching benefits, even beyond the community of neurodiverse learners. In the near term, AugmentedEF provides a tool that allows students with EF difficulties to improve their ability to independently complete STEM assignments, thus broadening participation in STEM learning. In the long term, AugmentedEF has the potential to become a general learning technology that improves outcomes for most students across practically any academic setting.
The project’s ultimate vision is for anyone to be able to augment their EF capabilities as easily as someone would put on glasses. AugmentedEF will serve as a model for innovative, scalable interventions that use assistive technology to support those who struggle with EF and attention issues to persist and thrive in a broad range of academic and workplace settings.
The primary goal of this project is to support the foundational capabilities for STEM for post-secondary learners with attention deficits and executive function (EF) needs. AugmentedEF uses emerging technologies to explore novel and accessible tools for teaching and learning.
EF represents a broad skill set that is central to STEM learning and academic success, including skills related to attention, persistence, emotion regulation, and inhibition control (Barkley, 2012). The marginalization of learners with attention and EF differences occurs throughout schooling, and is a further cause of rampant inequities in our education system. There is an increased prevalence of attention to EF issues such as ADHD in many marginalized communities, including communities of color (Miller, et al., 2009), low SES communities (Russell et al., 2016) and communities of English language learners (Liontou, 2019).
Given the demands placed on students in online learning contexts from the response to the COVID-19 pandemic, inequities due to EF issues have been brought to the forefront. Students with EF difficulties tend to underperform in independent work environments, like those required in many remote learning scenarios (Dahlstrom-Hakki et al., 2020), thus demands in digital environments disproportionately and negatively impact students with EF issues (Carter et al., 2020). While there are efforts to develop assistive technology to supplement a student’s organizational capabilities, many tools are expensive and exclusionary, and most rely on students’ ability to organize or initiate their own task monitoring (e.g., the use of a planner).
AugmentedEF presents a novel approach to this complex problem by developing an open-source learning tool that uses an augmented reality (AR) interface with a backend machine-learning architecture able to initiate and adapt task monitoring prompts based upon students’ perceived behaviors. AugmentedEF will be designed and tested with a broad audience of neurodiverse undergraduate students to examine and improve its efficacy as a STEM learning tool.
This tool represents a novel contribution to both the computer sciences and the learning sciences. AugmentedEF will develop an open-source tool based on machine learning algorithms that provides appropriate feedback to students related to their own level of focus and distractibility. The use of machine learning is an important feature because ultimately, it will not require a human to code off-task behavior.
There have been some initial attempts to look at user attention from the perspective of machine learning (Szafir & Mutlu, 2012) and others have attempted to explore prototypical ADHD behavior through the lens of machine learning tools (Duda et al., 2016). AugmentedEF will explore new algorithms and prompting tools that are useful to the field of EF support research.
Impact
The creation and validation of AugmentedEF is intended to have wide-reaching benefits, even beyond the community of neurodiverse learners. In the near term, AugmentedEF provides a tool that allows students with EF difficulties to improve their ability to independently complete STEM assignments, thus broadening participation in STEM learning. In the long term, AugmentedEF has the potential to become a general learning technology that improves outcomes for most students across practically any academic setting.
The project’s ultimate vision is for anyone to be able to augment their EF capabilities as easily as someone would put on glasses. AugmentedEF will serve as a model for innovative, scalable interventions that use assistive technology to support those who struggle with EF and attention issues to persist and thrive in a broad range of academic and workplace settings.
The creation and validation of AugmentedEF is intended to have wide-reaching benefits, even beyond the community of neurodiverse learners. In the near term, AugmentedEF provides a tool that allows students with EF difficulties to improve their ability to independently complete STEM assignments, thus broadening participation in STEM learning. In the long term, AugmentedEF has the potential to become a general learning technology that improves outcomes for most students across practically any academic setting.
The project’s ultimate vision is for anyone to be able to augment their EF capabilities as easily as someone would put on glasses. AugmentedEF will serve as a model for innovative, scalable interventions that use assistive technology to support those who struggle with EF and attention issues to persist and thrive in a broad range of academic and workplace settings.
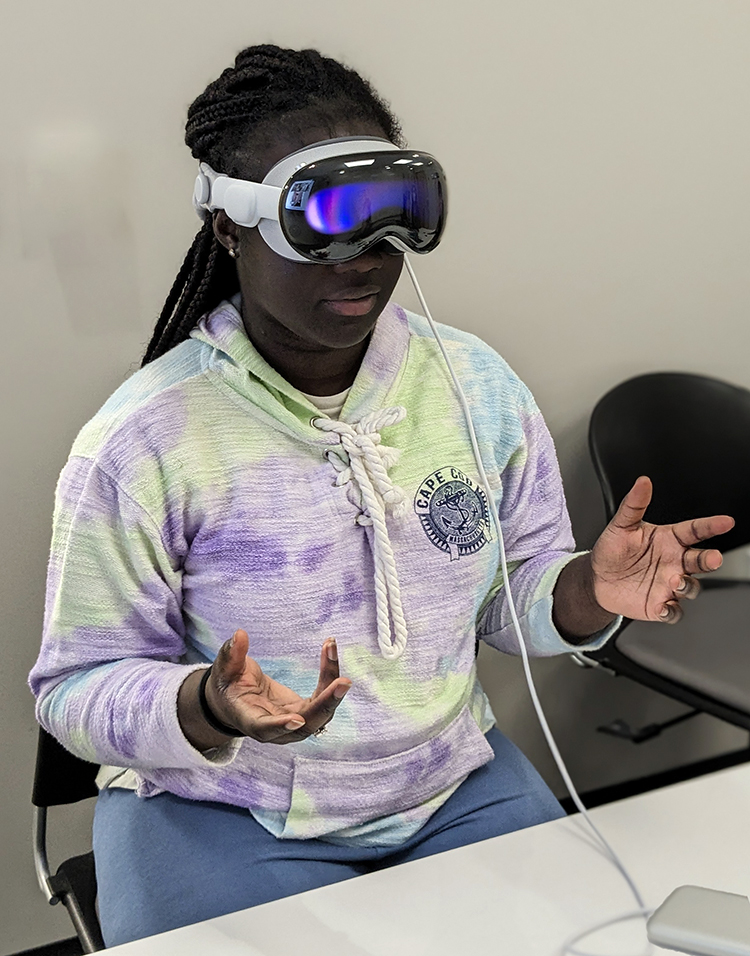



Funder:
National Science Foundation
Award Number:
2202291
Dates:
9/1/22 – 8/31/25
Share This Page: